The AI Revolution Still Needs Time
While AI has the real potential to become a business driver, Michael Hase from eco reveals how its success relies on data competence & process understanding.
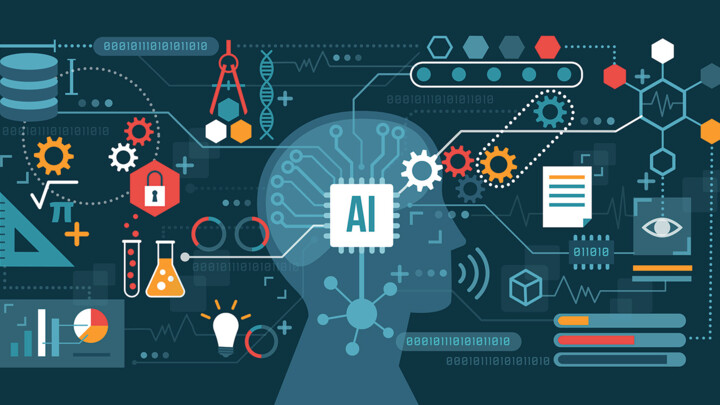
© elenabs | istockphoto.com
AI has the real potential to become a business driver. However, it will take some time to get there. Even though foundational models are widely available today, the development of AI applications is not about plug-and-play. What is needed are data competence and a clear understanding of processes.
Companies now have easier access to AI technologies than ever before. Specific software libraries are now so sophisticated that they enable developers to use AI models for certain tasks without in-depth knowledge of the underlying code or architecture. “AI can now be used as a regular software component, and this was simply not possible a few years ago,” explains Falk Borgmann, Head of Consulting at the service provider Deepshore in Hamburg. To avail of the models, no machine learning expertise is required.
Nevertheless, AI is still at an early stage of development, given that the creation of AI applications does not entail plug-and-play. “Very few use cases are covered one-to-one by pre-trained models,” Borgmann clarifies. What is therefore still needed is the competence of data scientists. This means that, from the multitude of models available, such data scientists must first select the model that best suits the specific use case at hand. Their task is also to fine-tune the parameters of the model with the relevant data so that it fulfils the particular purpose of the application. Alternatively, they can build a data persistence alongside the language model so that it only provides the semantics, but no content.
Such decisions require thorough analysis and must be made depending on the use case. From the foundational model to the finished AI application, there is still a lot of work to be done that requires human intelligence. It will, therefore, still take some time before the use of AI becomes commonplace in the economy. As of yet, many companies still lack vertical applications that support their specific processes. First and foremost, however, they need the necessary “AI readiness.” To achieve this, companies must establish data management and governance that fulfil the requirements posed by AI – not only in terms of data quality, availability, and security, but also in terms of ethics, regulation, and liability. Last but not least, users must decide for themselves whether they want to use AI models via service APIs or to deploy them locally. This latter approach requires more time and effort.
Breakthrough in three or more years
Analysts at Gartner expect AI to achieve a breakthrough in just a few years. They predict that, by 2026, more than 80 percent of companies worldwide will access language models via interfaces or use ready-made AI applications. Last year, this figure stood at just five percent. It is also expected that the increasing use of AI will be reflected in financial statements. According to surveys conducted by the investment bank Goldman Sachs, corporate decision-makers expect AI to become a factor that will have a major impact on their business over the next three to ten years.
The technological prerequisites for AI to truly become a business driver have been fulfilled. Not only have the foundational models become increasingly powerful in recent years, but the way in which they are used has also become more simplified. As a result, AI developers no longer have to start from scratch and train neural networks themselves, as pointed out by the Deepshore expert Borgmann. If a model already masters German vocabulary and grammar, for example, it ‘only’ needs to be introduced to the finer points of legal terminology in order to create a chatbot for lawyers and judges. “A much smaller and therefore more cost-effective task.”
Last year, Deepshore developed the prototype of such a bot. This is designed to support practicing lawyers by relieving them of time-consuming research tasks. The system can respond to questions on specific issues and provide initial legal assessments. In doing so, it refers to rulings that are relevant to the issue at hand. The bot takes on “the role of a well-trained legal clerk who assists a lawyer or judge,” explains Borgmann. It cannot replace the experience that a lawyer has gained over years of practice, but it does shorten the search for relevant rulings.
In the first release, the bot has been limited to labor law. However, it is intended to be gradually extended to cover other fields of law and to be further developed into a comprehensive legal assistance system. Specialist publishers who are in discussion with Deepshore have indicated their keen interest on this topic.
In creating the solution, the experts at Deepshore recognized two important factors. Firstly, they weaned off the bot from the so-called “hallucination” to which AI models are prone, meaning that the users should be able to rely on the system which doesn’t fabricate any rulings. Secondly, the developers placed great emphasis on the infrastructure independence of the AI application. They developed the solution as a cloud native application that can be deployed in any Kubernetes environment, such as AWS, Azure, or the Google Cloud Platform (GCP). The alpha version was launched on the infrastructure of STACKIT, the cloud of the Schwarz Group.
Support by the public sector
Many companies in Germany – service providers, software vendors, as well as users – are currently working on AI projects in a similar manner. The “Learning Systems” platform of the German Academy of Science and Engineering (acatech) lists around 1,170 AI applications. However, almost 80 percent of these are still in the development phase. Many of these projects are supported by the public sector. Since 2018, as part of the national AI Strategy, the German federal government and federal states have supported the establishment of more than 100 digital hubs and competence centers, which serve the transfer of knowledge from research to practice. One such transfer center is the Innovation Park Artificial Intelligence (IPAI) in Heilbronn, in which Deepshore is involved alongside companies such as Porsche, Schwarz, and Würth, as well as research institutions such as Fraunhofer IAO and TU Munich.
Since last year, more and more hyperscaler partners have been looking at the opportunities offered by cloud providers’ AI portfolios. Triggered by the hype surrounding ChatGPT at the end of 2022, AWS, Google, and Microsoft have significantly expanded their range of services and tools for generative AI. They now provide pre-trained foundational models via their platforms, which users can easily access via APIs. The British consulting firm Canalys sees huge business potential for service providers and software vendors who develop customized solutions for specific use cases or individual industries on this basis. Overall, analysts expect the global market for generative AI, including consultancy and service providers, to grow from 15.4 billion dollars last year to 158.6 billion dollars by 2028.
Among the AI projects that were initiated last year is a chatbot created for Audi by Storm Reply – the AWS unit of the service provider Reply. The knowledge management tool is designed to help employees of the automotive manufacturer to efficiently, quickly, and reliably find internal information. This could be project documentation, risk assessments, or contact details. Storm Reply created the application based on the AI platform Amazon SageMaker. The bot only provides answers that are of relevance to Audi. Furthermore, rather than generating false answers, it explicitly informs users when it cannot answer a question with certainty due to insufficient data.
Microsoft partner aConTech, part of the teccle group, is also working on AI projects. Among other solutions, the cloud specialist based in Fürth has developed a bot for the estate industry based on Azure OpenAI. The system answers tenants’ questions and can provide them with information on contractual matters and utility bills. It also provides assistance in the event of various damages and defects. Within certain financial parameters, the bot can assign a tradesperson to fix the problem for the tenant, and it only notifies a human when things become more complex.
As is the case with comparable bots, aConTech’s AI application can still be upgraded. The service provider targets smaller real estate companies that manage up to 200 residential units. “The system is not yet sophisticated enough to handle the complex processes of larger real estate companies,” concedes Stefan Zenkel, Managing Director at aConTech. However, the framework of the application is in place, and it is scalable in principle. As he notes, “We wouldn’t object to a major representative of the industry being interested in further developing the bot together with us.”
Suitable language model and relevant data
In another project, aConTech is also creating a legal bot. The system is intended to support investors in due diligence procedures by detecting legal weaknesses in contractual documents. According to Zenkel, the quality of such an application relies crucially on two factors: firstly, the selection of the right language model, focusing on how well it is suited to the application purpose in terms of its architecture and whether it is able to deal with the specific language of the target audience – in this case, the legal idiom. Secondly, according to the expert, it is essential to feed the pre-trained model for fine-tuning with relevant, high-quality data.
AI development sometimes requires a lot of perseverance, as shown by an ambitious project that Deepshore is currently driving forward. In a three-year research collaboration with the Technical University of Darmstadt, the service provider is developing an application that can be used to process mass data more efficiently – for instance, in the retail and financial sectors. The goal of the “ETL4Balance” project is to fully automate the process in which data from various sources is brought together, reformatted for further processing, and uploaded to data warehouses or data lakes. This process is known as Extract, Transform, Load (ETL).
ETL systems are usually operated by specialist staff who work around the clock to manage loads and capacities. Within the framework of “ETL4Balance,” the systems are intended to be empowered by reinforcement learning in order to autonomously plan processes, to activate additional resources as needed, and to respond to disruptions in real-time. Additionally, they should be able to anticipate upcoming requirements with precise forecasts to react at an early stage before an error occurs. This project is funded by the German Federal Ministry of Education and Research (BMBF) and supported by Schwarz Digits, the IT subsidiary of the Schwarz Group, as a commercial partner.
The project demonstrates one of the main benefits of AI: the ability to automate complex and labor-intensive processes, ultimately leading to enhanced productivity. A decisive factor here is the ability of self-learning systems to recognize patterns in large data sets and make autonomous decisions, taking a variety of factors into account. While access to the foundational technologies is easier today than ever before, developing AI solutions for specific use cases remains a challenge. This requires significant technical skills in handling data, as well as a deep understanding of the processes to be optimized. This is unlikely to remain unchanged in the future.
“AI in Practice”
With the “AI in Practice” initiative, eco – Association of the Internet Industry promotes the development of AI applications in SMEs. Best practices and recommendations for action are designed to assist companies in unlocking the potential of AI. The initiative focuses on manageable concepts. In addition to AI applications, other key topics include: platforms for AI offerings, ecosystems for data exchange, sustainable operating concepts for data centers that process AI workloads, and regulatory frameworks for AI use.
As Manager EuroCloud, Michael Hase is responsible for coordination and further development of cloud topics at eco. He joined the association in November 2023. Prior to that, he worked for more than 20 years as an IT journalist and wrote for industry magazines such as IT-Business and CRN. His focus was on the ecosystems within the IT market.